COVID-19 presents an unprecedented predicament: Every day, leaders must make momentous decisions with life or death consequences for many—but there is a dearth of data.
In this interview with Impakter, Oded Netzer – a Columbia business professor and Data Science Institute affiliate who builds statistical and econometric models to measure consumer behavior that helps business leaders make data-driven decisions – discusses how leaders from all fields can make sound decisions with scarce data to guide them.
How can leaders, regulators, and businesses make informed decisions with scant data on COVID-19?
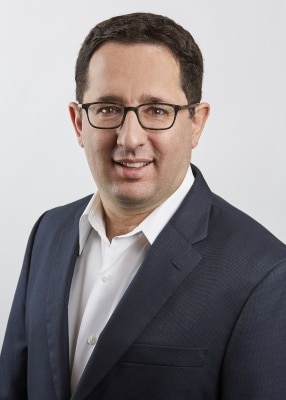
Oded Netzer: For those of us with an expertise in data science, the COVID-19 pandemic has been a humbling experience. In the past few years, we have been promoting the notion of data-driven decisions and encouraging decision-makers to use the wealth of data typically available to them to make better and more informed decisions.
We have been encouraging leaders to use rich historical or comparable data to estimate a sound model and identify repeated patterns, and then apply these techniques to guide their decision making.
How has the coronavirus upended that conventional thinking?
Oded Netzer: Unprecedented realities, such as the one we are facing now with the COVID19 pandemic, provide a challenge to this traditional practice of data science. In such situations, we have very limited historical or benchmark data to base our decisions on.
Hence, we need to combine the limited data we observe, which are often far from perfect, with a good amount of intuition and domain-specific acumen. In a course I teach at Columbia Business School with colleagues Christopher Frank and Paul Magnone, we talk often about quantitative intuition—a combination of data science coupled with a leader’s sound judgment and acumen.
What about using comparative coronavirus data from other countries or previous epidemics?
Oded Netzer: When evaluating the expected pattern of COVID-19 diffusion in the U.S., we often use data from other countries that may be at more advanced stages of the pandemic diffusion (e.g., China, South Korea, or Italy). Similarly, we use data from related epidemics such as SARS, MERS, and even from the Spanish Flu of 1918.
Obviously, none of these related data sources can be directly applied to the current U.S. epidemic. Using data from other countries is difficult because countries differ with respect to their political regimes, population-age distribution, health care systems, etc. Differences such as population-age distribution can be fairly easily handled in a model, but adjusting for aspects such as political regimes and privacy concerns is much more difficult.
Similarly, given the limited information we have on the COVID-19 virus, it is difficult to assess how similar or different this virus is to previous epidemics. These challenges of using the limited data we have, doesn’t mean that we should throw the baby out with the bathwater and ignore these possibly useful data altogether. We cannot expect a model to directly tell us how to adapt previous data to the current situation. This is where we need to pour in some researcher/human judgment and domain acumen.
Are the comparative data similarly challenging in the field of business?
Oded Netzer: Yes, in terms of business and economics, the question arises how much can we learn from previous financial crises to predict the financial implication of the current pandemic and our ability to recover from it. Clearly, one cannot directly use the same data or model from 2008 and apply it to our current financial predicament, but at the same time, there is a lot we can learn from the past.
RELATED ARTICLES: Columbia University Researchers Use AI to Develop Treatment for Coronavirus |Brexit Amid COVID-19: Can Britain’s Financial Services Industry Breathe? |How Individuals Can Take Action During the COVID-19 Crisis |COVID-19: Link with Air Pollution? Italy’s and China’s Experience |Compared to the Climate Emergency, COVID-19 Looks Like a Walk in the Park
So how can data scientists and leaders make good decisions during this crisis?
Oded Netzer: Use judgment and acumen of experts. Judgment and expertise may be used to adapt models and data from other domains to the current situation. Machine learning, statistics, and econometrics are useful to derive information from existing data and predict the future as long as the environment is similar to the environment used in the data analysis.
Human judgment and theory can guide us in deciding which factors are similar or different across datasets or situations, and how one can adapt or combine different sources of data to the current situation. Humans are good at pattern recognition; computers are good at data processing. At times like these, when data are limited, we need to combine both.
Use worst, base, and best-case scenarios. We have seen many scientists use worst, base, and best-case scenarios when presenting predictions for the COVID-19 pandemic. When uncertainty is high, it is useful to present alternative scenarios to allow decision-makers to appreciate the uncertainty involved in the situation.
Presenting alternative scenarios is also useful to plan for the worst-case (rather than base) scenario (e.g., number of ICU beds and ventilators needed). When building the scenarios, the benchmark data, even if not fully comparable to the current situation, can be used to define worst or best-case scenarios (e.g., taking Singapore or Japan epidemic curves as proxies for best-case scenarios).
Use simulations. For complex situations, where actual data is limited, one can use a simulation to simulate alternative scenarios. Rather than predicting a specific number, as is often done in many machine learning models, simulations allow the researcher to explore the impact of different parameter values, which the researcher may only know with uncertainty, on the expected outcome. For example, one can simulate the spread of the epidemic under different assumptions of how many people a COVID-19 positive patient infects.
Synthesize information. Whereas each of the benchmark datasets may be incomplete and inadequate to the current situation, synthesizing the information across datasets may be useful in getting a clearer picture of the situation as a whole. Again, the task of synthesizing information is best done by humans rather than machines and calls for quantitative intuition.
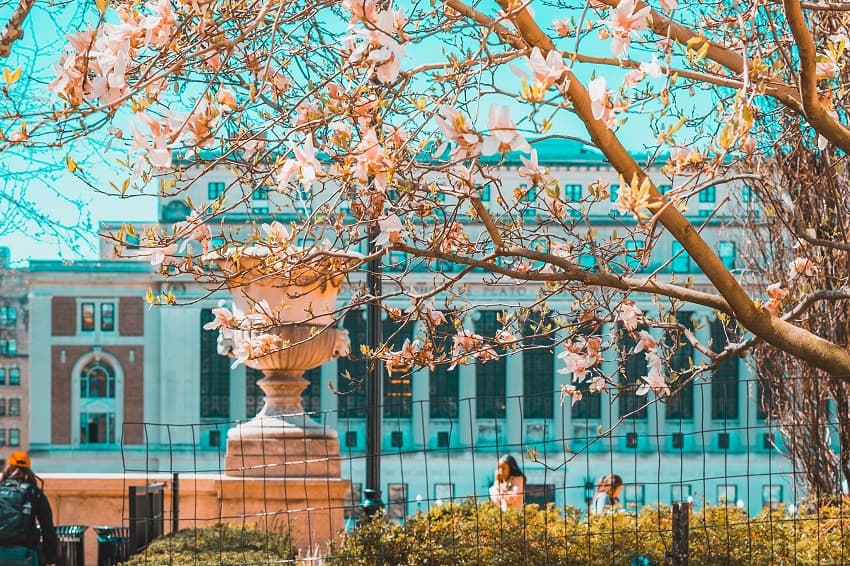
Are you optimistic?
Oded Netzer: As more data become available in the U.S. and around the world, and as we learn more about this unique pandemic, our confidence in the models and data-driven decision making will increase, and with it our ability to manage this pandemic.
Thus, by “flattening the curve” we are not only buying time to avoid overburdening our health system and finding a treatment for this dangerous virus, but we are also acquiring more and better data that will allow us to make better data-driven decisions in fighting and managing this unprecedented pandemic.
You’ve said the Data Science Institute at Columbia is a definitive example of an interdisciplinary hub for researchers to conduct data-driven research. How will such an entity be helpful during this pandemic?
Oded Netzer: Absolutely, the Data Science Institute is a great example of a true interdisciplinary center that brings together great minds from across Columbia University, and the Institute also prides itself on using “data for good.” The COVID-19 pandemic has affected almost every aspect of our lives from medicine, to public health, to social well being, to economics and business.
Addressing this major shock to the world requires an interdisciplinary effort. At the heart of quantitative intuition is synthesizing the limited data we have with expertise, intuition, and judgment. Particularly at the early stages when data about the pandemic and its effects are sparse, interdisciplinary teams such as the ones at the Data Science Institute can offer expertise, intuition, and sound judgment to complement the limited data we have and make the most of it.
In the cover picture: Global Leaders in a meeting. Photo Credit: Wikipedia
Editor’s Note: The opinions expressed here by Impakter.com columnists are their own, not those of Impakter.com